In the previous Part 1 of the interview, we met with Geonuk Kim of the AI technology team, who won the Most Innovative Prize for the ‘visual prompting-based one-shot defect segmentation deep learning technology’ at the ECCV (European Conference on Computer Vision) 2024 Challenge, an international conference on AI and computer vision. In this Part 2 of the interview, we asked him about the reason and process for researching this technology, as well as the insights he gained from ECCV 2024 and his future plans.
* Go to Part 1 of the interview with Geonuk Kim, winner of the ECCV 2024 Challenge
“I feel very rewarded that my visual prompting technology has been recognized by the world’s best AI conference”
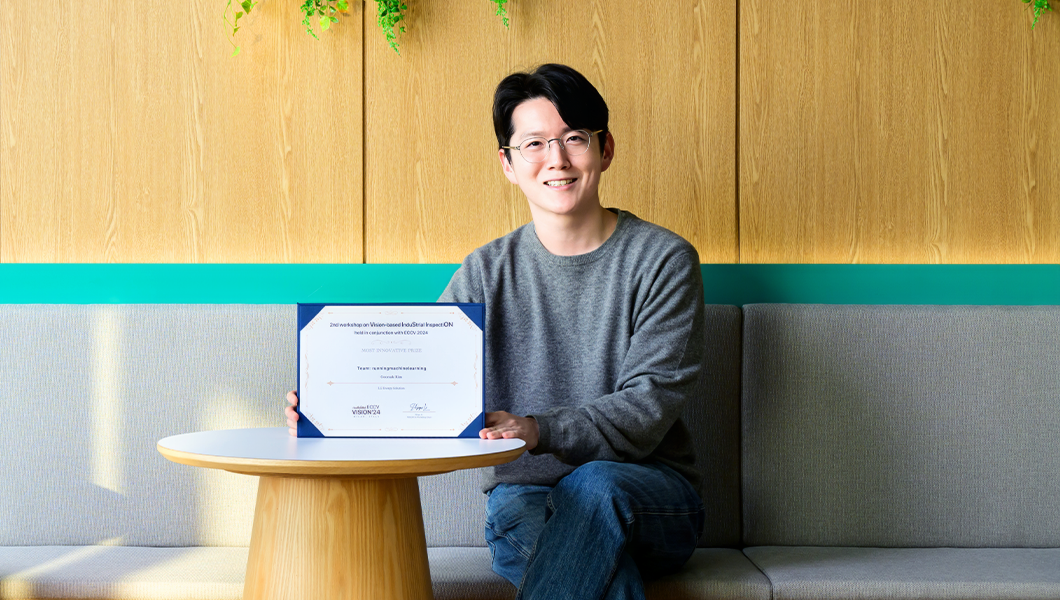
The AI technology that Geonuk Kim proposed at the ECCV 2024 Challenge was the visual prompting-based one-shot segmentation deep learning technology. Visual prompting is one of the deep learning technologies that recognizes objects based on the prompts fed by the user.
Winning the Most Innovative Prize through successful research, Geonuk Kim said that he was filled with emotions to have the opportunity to present his research results at the conference by himself after completing his master’s degree. Geonuk said, “I am really glad that the ECCV recognized my efforts to technically solve the difficulties I had while carrying out the projects.”
Research journey to winning the Most Innovative Prize at ECCV 2024!
Geonuk Kim has gone through numerous research projects to reach the top of the ECCV 2024 Challenge. What prompted him to research visual prompting deep learning technology? We met Geonuk to dive deeper into his story.
Q. What prompted you to research visual prompting deep learning technology?
While conducting data labeling work, I felt the need for visual prompting deep learning technology. Data labeling is a very significant task when it comes to general deep learning technology. In order for AI to learn a large amount of data, labeling must typically be done first. At that time, I was in charge of vision inspection to detect defects after the package welding process to weld the cathode and anode. As I had to analyze and assign labels to over 300,000 sheets of data, I felt so much difficulty because the amount of data to be labeled was so large.
An even bigger problem that required a solution was that defective data often got mixed with non-defective data due to the generation of new types of defects or changes in defect criteria. It is practically impossible to detect new defects and label them by looking at numerous defectless data with the naked eye. This is why I tried to come up with a way to automate vision inspection and improve the accuracy of defect detection, which led to an idea to utilize visual prompting technology after much thought into the issue.
Q. What efforts did you make to advance this technology during your research?
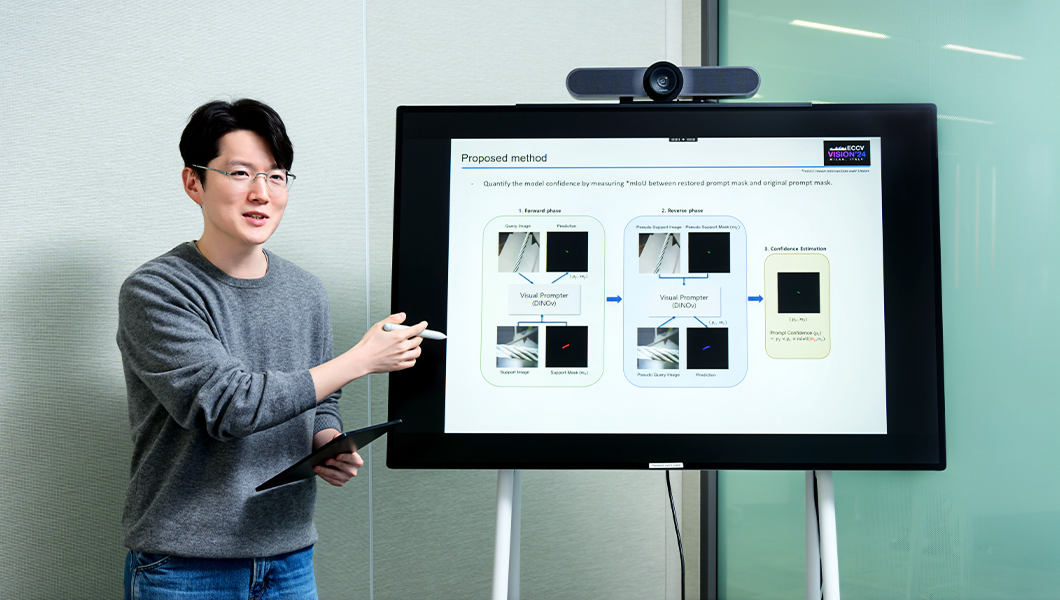
In the field of software, there is the open source culture where source codes are made public so that anyone can use them. Thanks to this development culture, advanced methodologies are continuously being derived based on the open source codes.
When I started researching visual prompting technology, I could not use the already existing source code because it was incomplete. I referred to the paper to make thorough comparisons to see if I had missed anything. Through this process, I was able to improve the completeness of the visual prompting technology and actually implement it.
Q. What are some aspects that helped advance your research?
It took me a lot of time to learn the methodology of visual prompting technology, with the particular challenge coming from designing a methodology. I often had technical discussions with Kangil Lee of the AI Technology Team, who then suggested the idea of using a deep learning model that can be applied without training. Many things, including this idea, helped me in advancing my research.
In addition, we share ideas about various tasks with each other at weekly team meetings. And one time I was greatly inspired by the task to develop an AI to design the optimal cell, that was being researched by one of my teammates. The task was different from the existing method of inputting attribute values of cell design and outputting design factors. The proposed task was about outputting the attribute values of cell design by inputting design factors. What I found particularly impressive was the methodology to convert the direction to ‘Y to X,’ from the existing focus on the ‘X to Y’ order.
Towards the advancement of AI technology! What are the research achievements and future path?
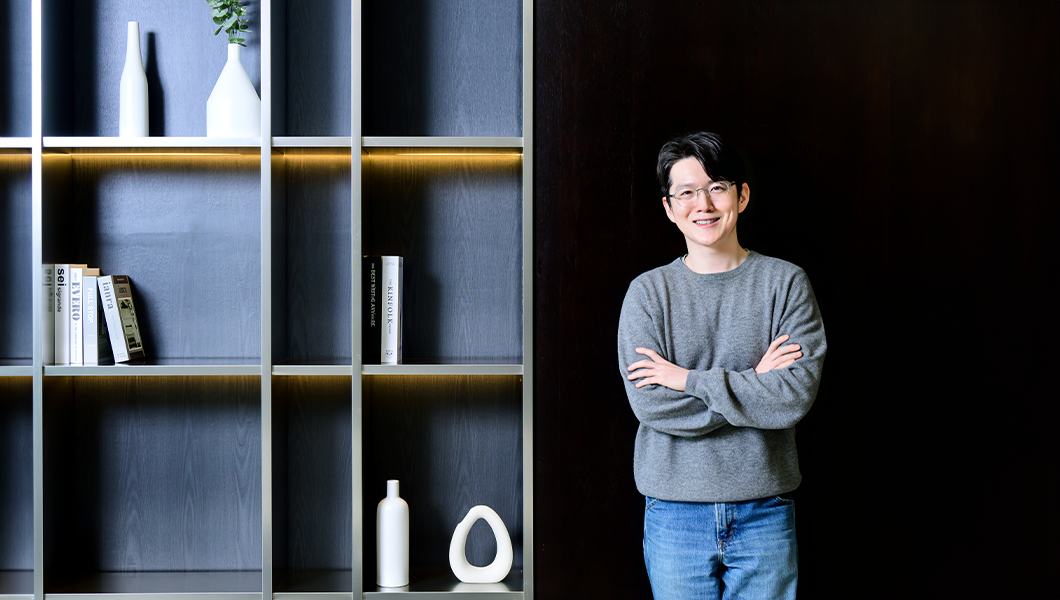
Geonuk Kim is so passionate about technology research that he participated in ECCV 2022, as well. Thanks to his passion, he was able to achieve significant research results in the field of artificial intelligence.
Geonuk Kim expressed his will not to rest on his laurels but to move forward based on the insights he gained from ECCV 2024. His biggest gain from participating in the conference was the fact that he established his research direction. There are still many unresolved challenges in the field, such as domain change, borderline samples, class imbalance, etc. Geonuk said that he gained great courage by sharing his difficulties with industry experts and was able to gain confidence in what kind of research he needs to conduct in the future.
Q. What progress have you made in the field of AI?
In regards to AI development, sufficient learning data must be secured while managing them is also very critical. LG Energy Solution can accumulate large amounts of data as it is continuously producing batteries. At the same time, it also needs a system that labels such data efficiently and automatically.
This is how I came up with ‘active learning’ technology after developing visual prompting technology. Active learning is a technology in which an AI model directly labels newly acquired data. In particular, active learning technology directly infers and labels data that are updated every month based on what the model has previously learned. The advantage comes from the convenience that the user only needs to assign labels to data that cannot be judged by the AI model. Following this process, the work time was reduced by about 75%.
As such, I feel a great sense of accomplishment as a computer engineer when the AI model I created does not only remain in a paper or code but actually solves a problem in the field.
Q. What research will you conduct at LG Energy Solution in the coming years?
Although visual prompting brings great innovation to data management, the processing speed slows down when the amount of computation increases. Since more work can be assigned to this model if the computation speed is increased, I am going to optimize it. I am currently trying to compress the visual prompting model to make it lightweight.
I also plan to research AI technology to realize a smart factory model, which is the goal of our AI Technology Team. We are utilizing AI not only in the visual inspection that I am researching but also in cell design, R&D, etc. There is a project that aims to drastically shorten the time for cell design and one that aims to preemptively determine lithium deposition using battery charge/discharge data. In this way, the AI Technology Team plans to use AI as a tool to automate the entire process of battery manufacturing from development to production by applying AI technology.
Q. Lastly, do you have any words to say for our readers?
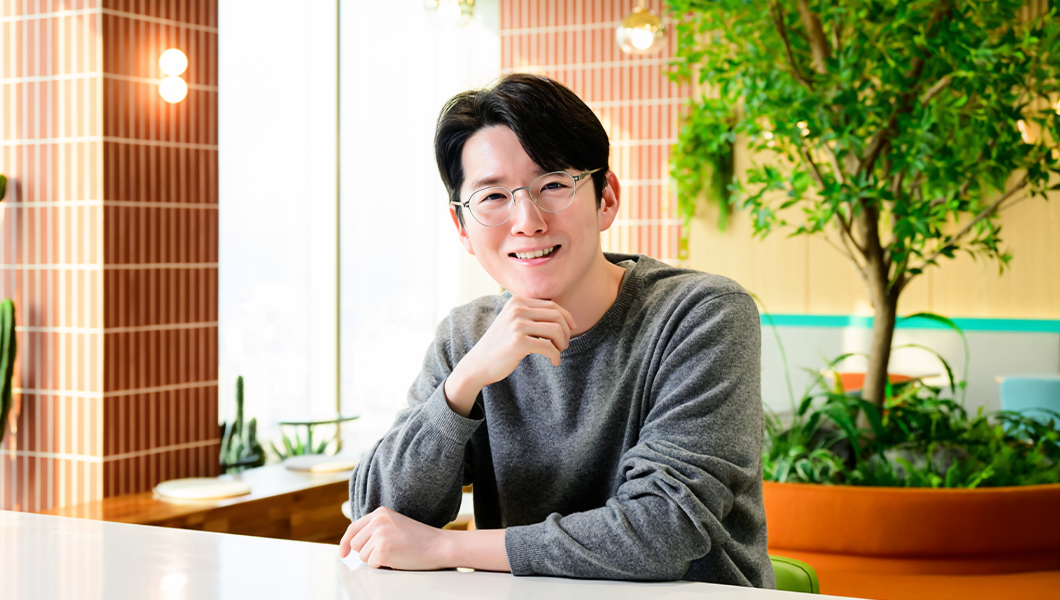
I was able to come up with a small innovation from conducting simple and difficult work. If my experience can inspire someone and help with follow-up research, that alone is a great reward. I hope that AI will develop through a series of processes to provide humans with a freer and more convenient life.
Lastly, I think LG Energy Solution can be a good opportunity for those who want to join the growing battery market. I will work with them to help LG Energy Solution leap higher.
So far, we have met Geonuk Kim, the winner of the ECCV 2024 Challenge, through the two parts of his interview! We were able to listen to his story about learning and researching visual prompting deep learning technology to solve the challenge in his work, as well as his future vision.
Geonuk and other members of LG Energy Solution are fired up with enthusiasm about technological innovation. We expect that these efforts will converge and contribute greatly to creating a convenient and happy future for all of us!