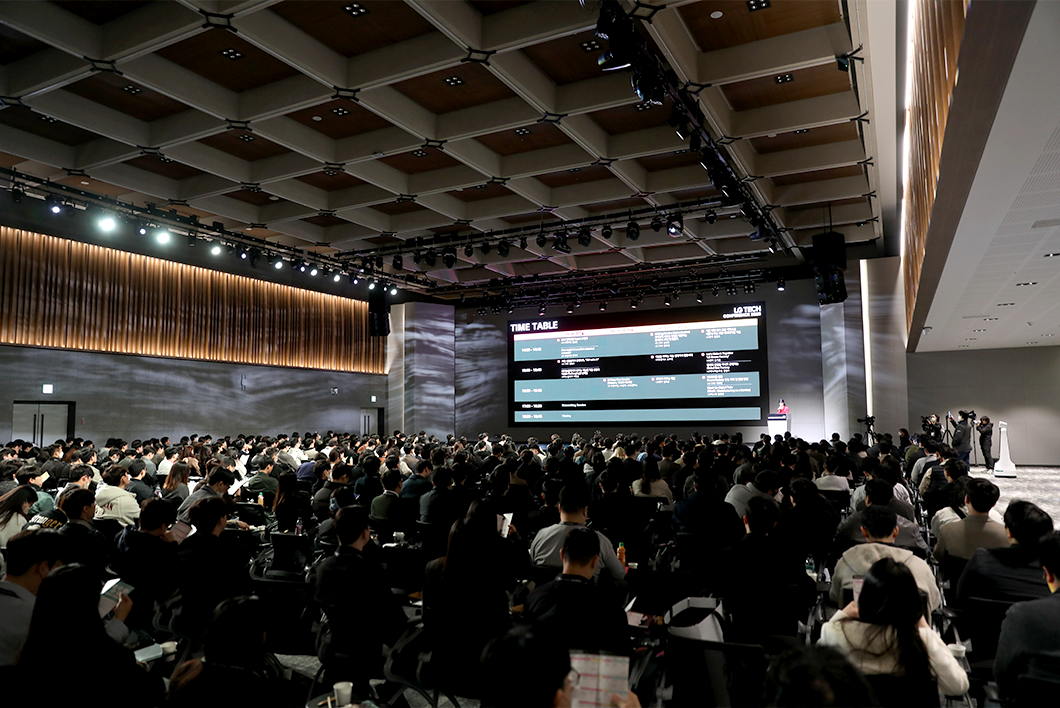
The LG Tech Conference was held on March 16 at LG Science Park in Magok, Gangseo-gu, Seoul, to attract outstanding R&D talent. LG held tech sessions in a total of 26 technology fields, including AI, big data, metaverse, blockchain, and smart factories, and introduced LG’s best practices. A session piqued the interest of top R&D talent against the backdrop of rapidly growing AI, as represented by the emergence of Chat GPT, Stable Diffusion, Dali, and other technologies. Let’s take a closer look at Ji-soon Choi’s presentation from the specialized session, whose team developed automotive battery safety diagnosis technology using AI.
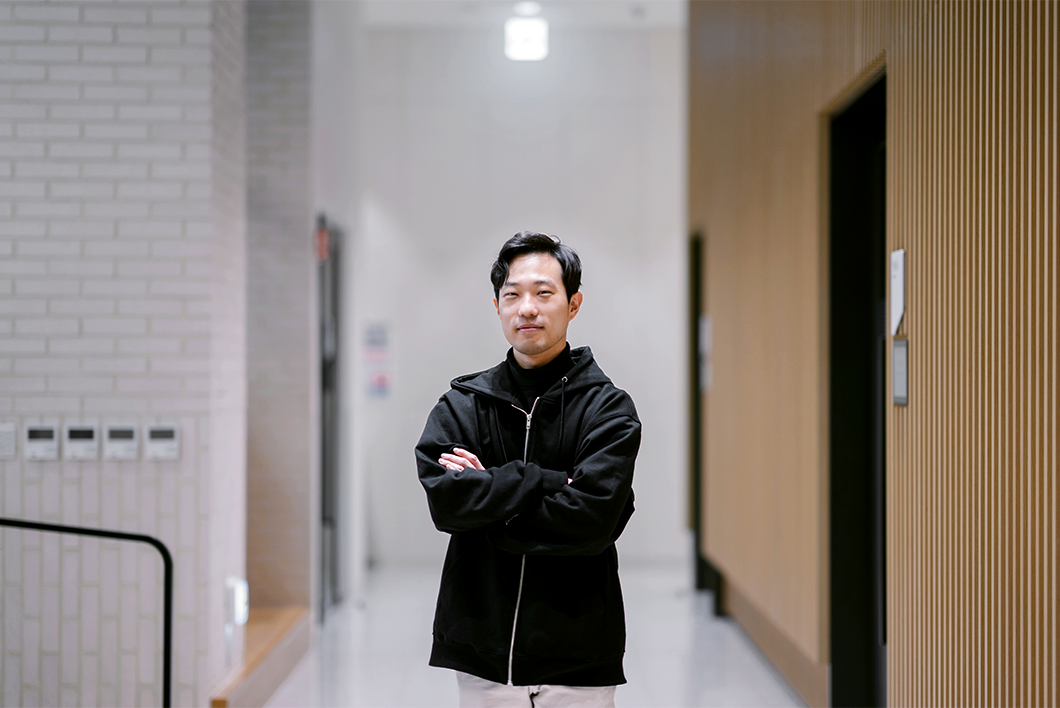
Will Artificial Intelligence Outperform the Diagnoses of Medical Specialists?
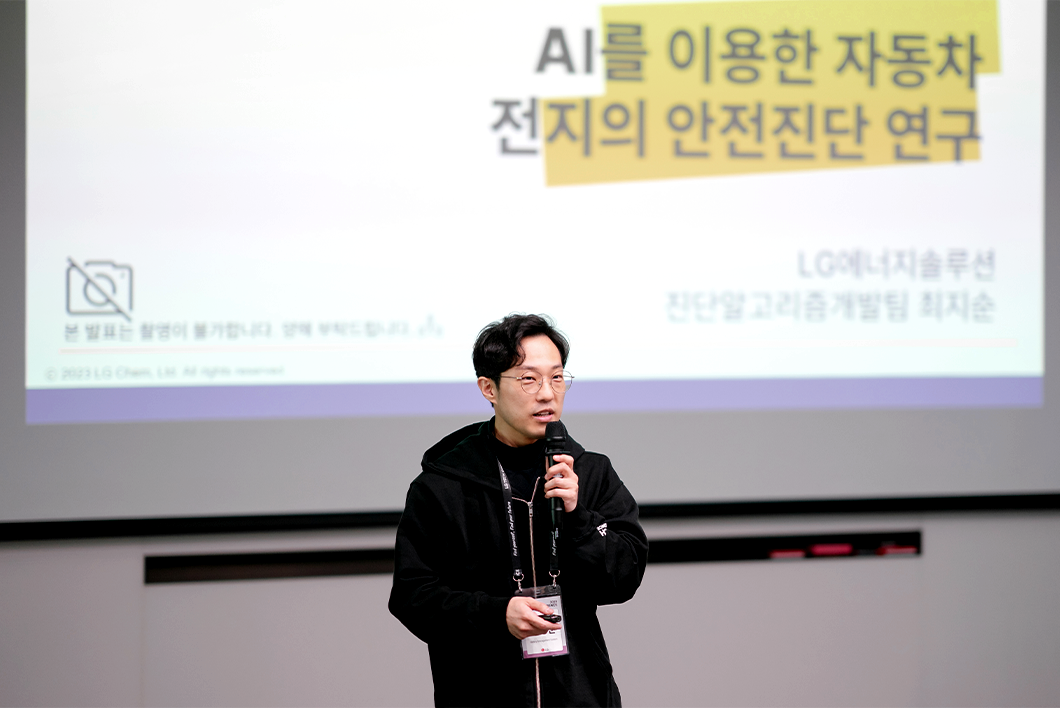
With regard to reading medical diagnoses, AI has already surpassed some experts. When 2,364 hip joint X-rays were used to diagnose the presence or absence of fractures, the specialist’s accuracy was 77.5%, while the AI was 92%. The specialists employ the cross-diagnosis method. For example, if the first specialist fails to judge an image, it is passed on to the second specialist. This continues until the fifth round.
AI, on the other hand, has achieved higher performance at a faster rate using simpler neural network models. Elderly hip fractures should be diagnosed and treated within 48 hours. As a result, artificial intelligence will become increasingly important in the healthcare industry.
Can Artificial Intelligence Outperform Experts in Assessing Battery Safety?
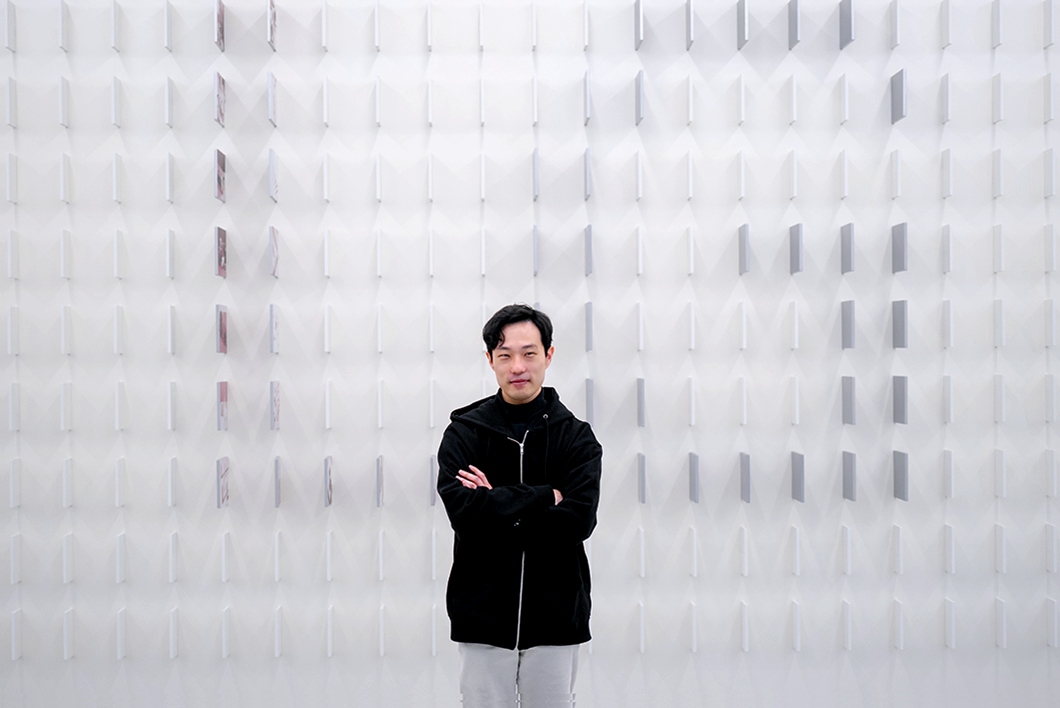
Experts at LG Energy Solution examine the battery signal recorded on the monitor in the same way that a doctor examines a CT image, looking for feature points and patterning the observed defects. Can AI, as in the previous case, outperform experts in battery safety diagnosis? Using 3,000 battery data points, AI and experts diagnosed defects with 81% accuracy and 71% accuracy, respectively. With limited data, AI could not match the level of expert diagnosis. However, when the data quantity was increased to over 8,000 data points and more complex patterns were added, the potential of using AI technology was confirmed.
Batteries can fail due to a variety of electrochemical or mechanical factors. Furthermore, a precise diagnosis can reveal the type of defect or level of deterioration in the specific materials that comprise the battery. However, if the previous method analyzes primarily in a human-readable format, AI can make decisions even with general raw data without special preprocessing, similar to how it can tell the difference between dogs and cats using images. Each pixel in a picture contains judgment data. However, just as AI recognizes objects by focusing on prominent features, such as a cat’s eyes or nose, it can also determine whether a battery is normal by connecting data from a specific area to a material defect.
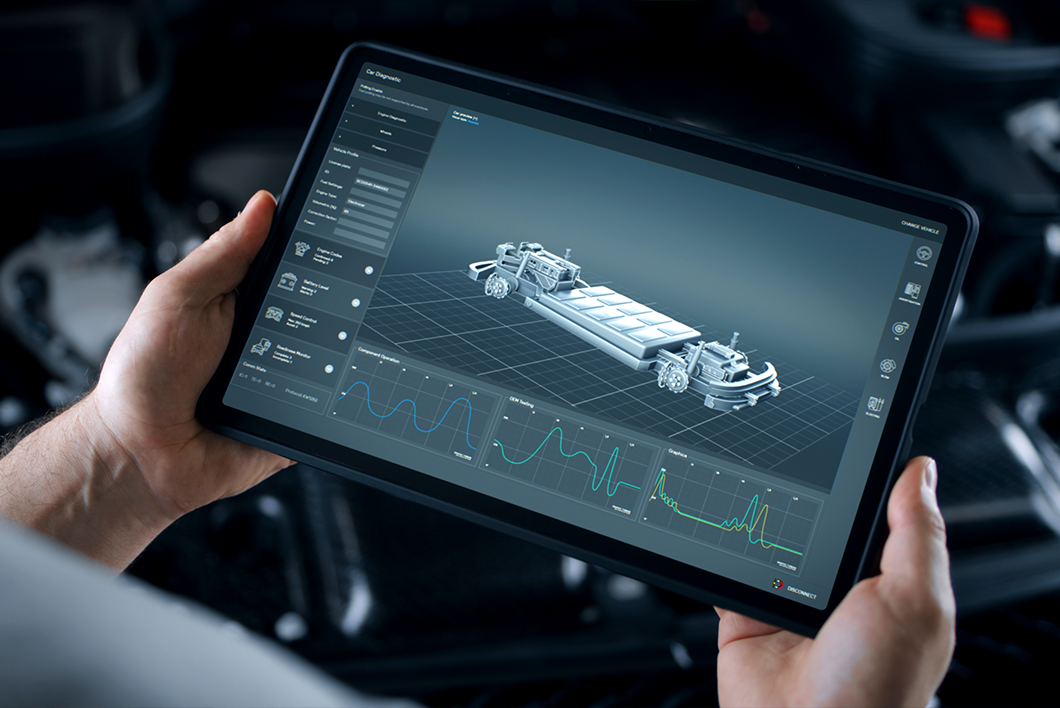
AI technology can also be used to assess the performance of batteries. A cell’s performance can be determined by factors such as capacity, output, heat, safety, and rapid charging. Previously, specific human-developed experiments were used to assess cell performance. However, using big data analysis, AI can efficiently perform comparable tasks in a typical driving environment.
LGES is enhancing its specialized skills through active collaboration with various universities and internal research. It has been working with Harvard for three years. The collaborative research project involves converting data from battery charge, discharge, and degradation into images. In Korea, LGES is collaborating with KAIST on a 2D CNN model to create a network that predicts the point at which battery capacity drops sharply at an earlier time.
What Is the Procedure for Determining the Safety of a Car Battery?
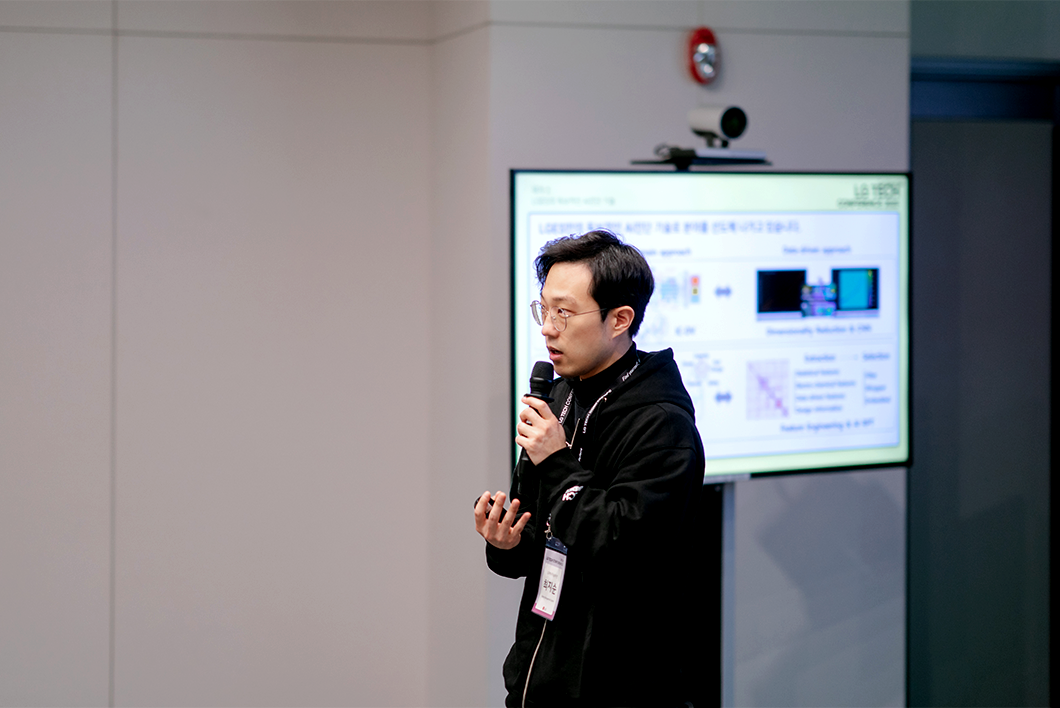
The safety of an automotive battery can be determined in five steps. First, we generate input data through experiments. Input data includes results from common cycle tests and precision experiments performed under standard conditions. Following the cycle test, the output is obtained via a labeling process. Cells are disassembled and analyzed in order to detect various types of defects and match specific observations to specific batches.
Following that, the relationship between input and output is derived. This step includes indicator extraction and model learning. Through various big data analyses, machine learning learns to distinguish between normality and abnormality by examining statistical and DX indicators. To commercialize this process beyond research, an MLOPS (Process Suitable for Machine Learning) system that can automate repetitive tasks and build a library in a standardized form was also developed so that the model can be applied to the site.
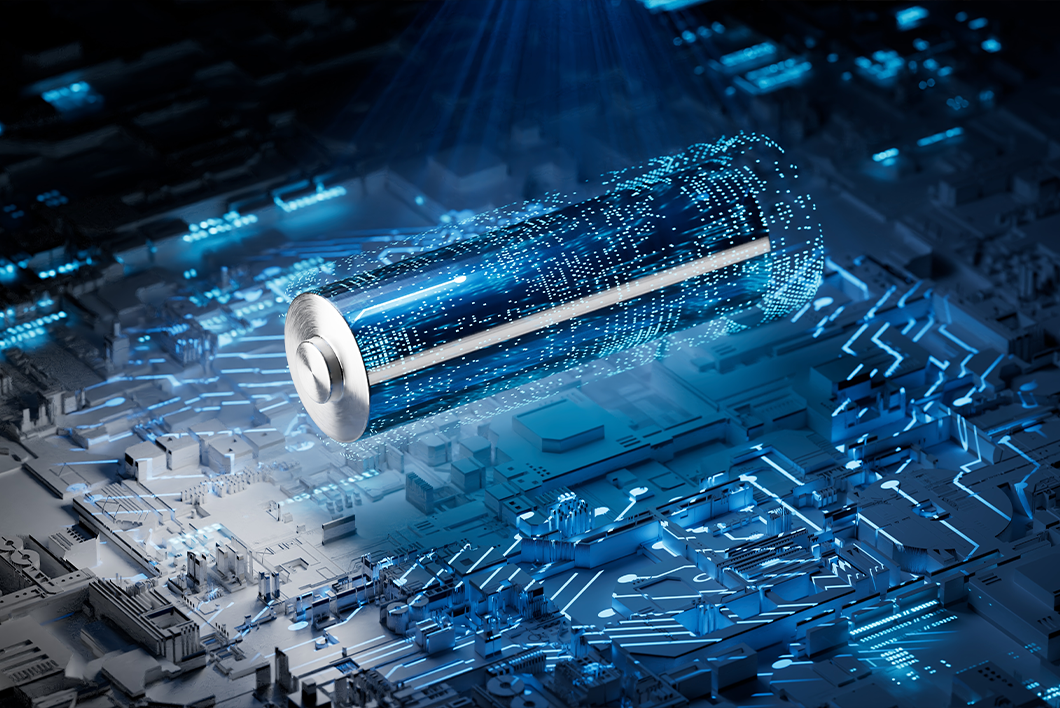
Previously, when approximately 3,000 battery data and a simple driving pattern were provided, the AI was not as precise as experts. However, when this data set was expanded to 8,000 data points and a slightly more complex pattern was used, AI achieved 78% accuracy while human experts achieved 52%. It can be concluded that AI can outperform humans. However, understanding how and why the AI diagnosed it in the manner that it does remains a challenge. This process is expected to be as difficult as humans attempting to understand AlphaGo’s moves. However, we are nearing the conclusion that it is possible to achieve a high level of diagnostic performance by first defining the range of voltage data that must be focused on when analyzing and second considering multiple voltage areas rather than one.
AI Diagnostic Technology That Expands to the Cloud
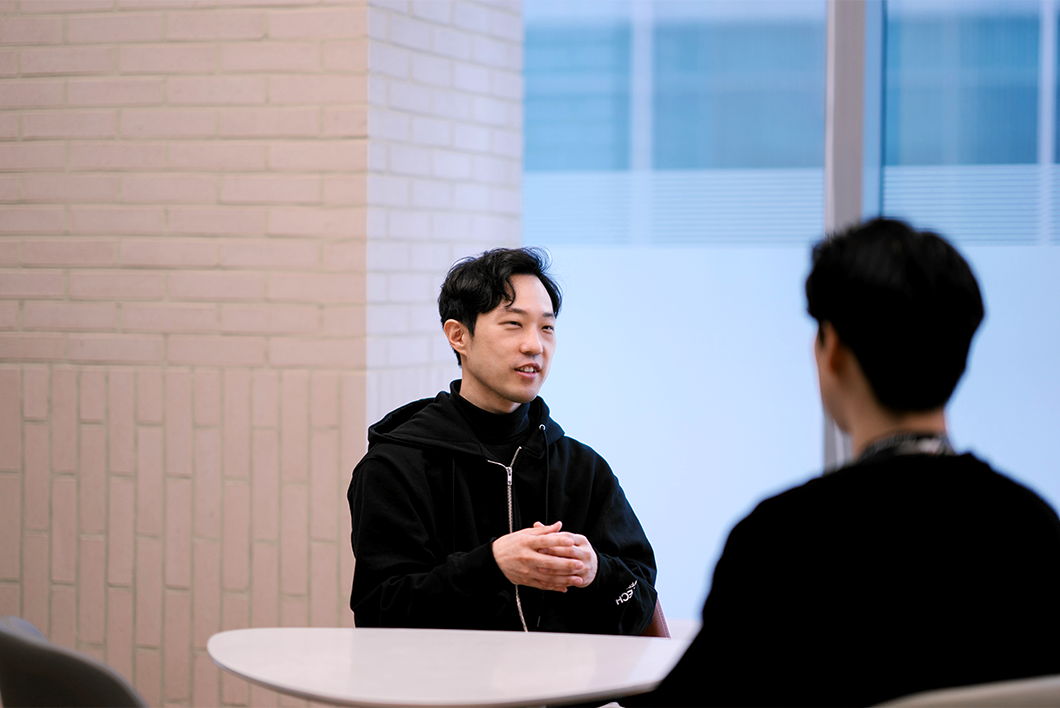
LG Energy Solution is expanding the range of safety diagnoses to the cloud using AI technology. Currently, the safety diagnosis is installed in the vehicle’s BMS (Battery Management System). However, the chip is too small to hold AI. Its computation and memory are limited. This is why cloud-based BMS is being considered. In addition, when the cloud BMS is introduced, more immediate and effective actions can be taken because the customer does not have to visit the service center. In addition, LGES is also preparing to update the diagnostic software for high-precision measuring devices in service centers.
Suppose LGES acquires technologies in these three areas: artificial intelligence, cloud BMS, and instant updates at the service center. In that case, it will be able to dominate the industry by gaining a competitive advantage over rival battery manufacturers.
To develop valuable and meaningful technology
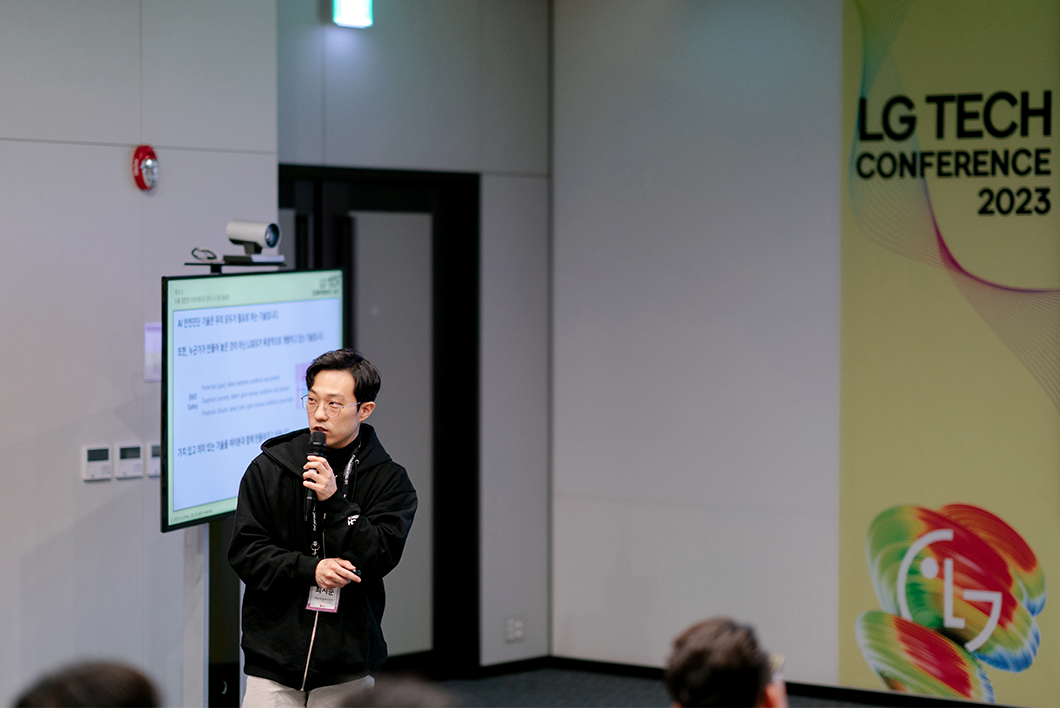
Moreover, we learned the value of safety diagnosis technology while doing this research. It is a technology that is required not only by individual companies or industries but also by society as a whole. Also, LGES is not following a ready-made path but developing original technology.
BMS is responsible for safety in electric vehicles. Previously, safety was primarily about protection. So the main tasks were to use software to keep the vehicles within the Safe Operating Zone and to create a hardware control device to do so.
However, even in this Safe Operating Zone, accidents can happen. Thus, LGES defines BMS safety as detection. Detection includes both diagnosis and prognosis. Diagnosis is identifying current defects, and prognosis is predicting future defects. The technologies required to perform this, including AI technology, are difficult to obtain and require ongoing research.
LGES is utilizing valuable technology to create an innovative future. We want to shape the future with exceptional R&D talent.